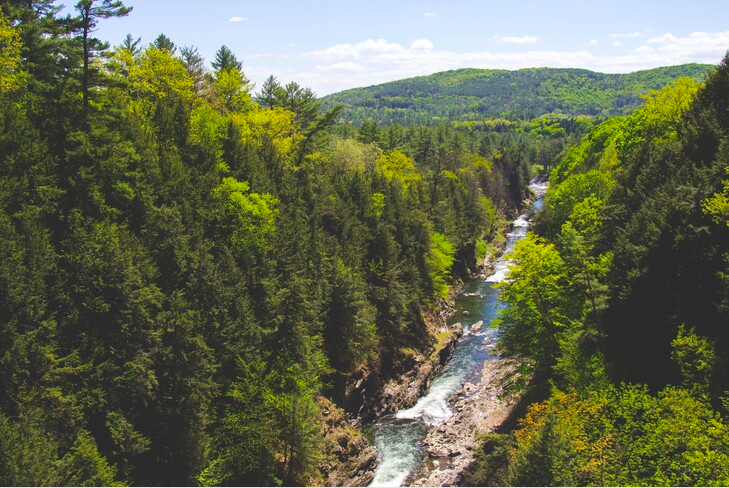
Streams contain a lot of information that can help scientists and environmentalists. But this information can be extremely difficult to analyze because of its complexity. A team of Penn State-led researchers said that their new use of a machine learning algorithm could help these scientists better understand these complex systems. (Image: Michael Browning/Unsplash)
Algorithm helps probe connections between stream chemistry and environment
Posted on August 3, 2021UNIVERSITY PARK, Pa. — Machine learning techniques may help scientists better understand the intricate chemistry of streams and monitor broader environmental conditions, according to a team of researchers.
In a study, the researchers report on the novel application of a machine learning algorithm to analyze how the chemical makeup of streams changes over time, particularly focusing on the fluctuations of carbon dioxide in the delicate and complex stream chemistry.
They added that scientists may be able to use the algorithm to study the role streams play in sequestering carbon dioxide and releasing it back into the atmosphere. Understanding this process is important because of the impact this greenhouse gas has on global climate.
“The chemistry of streams changes with time and as it changes with time, it can offer us a lot of information,” said Susan Brantley, distinguished professor of geosciences at Penn State and an Institute for Computational and Data Sciences affiliate. “Streams also have information about how carbon dioxide is being pulled out of the atmosphere, or pushed back into the atmosphere by a variety of processes. So, when we look at stream chemistry changing with time, we can learn more about carbon dioxide going in and out of the atmosphere, related mostly to natural processes, but also to some extent with processes that humans cause.”
The study also showed the relationship between rock chemistry and stream chemistry, said Andrew Shaughnessy, doctoral candidate in geosciences and first author of the paper.
“We found that the streams behave very similar to the way that the rocks behave,” said Shaughnessy. “So, we can use this process — this interplay between stream chemistry matching rock chemistry — that is happening today to infer these long-term processes.”
Among their discoveries, the researchers found that acid rain — which is unusually acidic rain or other forms of precipitation — reduced a watershed’s ability to sequester carbon dioxide. For example, sulfuric acid in acid rain could dissolve silicate materials in the watershed, which then affects the carbon dioxide sequestration process.
The challenge of monitoring stream chemistry is its complexity, which is why a machine learning method can be so valuable, said Shaughnessy. The rich complexity of streams is a bit of a two-edge sword, however, he suggested.
“The good thing about streams is that they integrate a lot of different processes, so you can measure the stream chemistry and learn about them,” Shaughnessy said. “The problem with streams is that they also integrate all these things. There are a lot of sources of solutes in the stream and the big challenge is being able to take the stream chemistry and separating all the different sources of the solutes to be able to learn about individual reactions taking place. Part of this project was reading the stream chemistry in terms of these mineral reactions.”
Prior to this method, researchers relied on a method called endmember mixing analysis, or EMMA, to interpret the sources of makeup of the stream, but variations in stream concentrations and discharges remained difficult to explain.
Machine learning can help unravel some of that complexity, according to the researchers, who reported their findings in a recent issue of the journal Hydrology and Earth System Sciences.
The team developed their model based on an unsupervised learning model called on-negative matrix factorization, or NMF. The model has also been used to understand complex relationships in fields as diverse as astronomy and e-commerce. As its name suggests, unsupervised learning is a type of machine learning that can find patterns in data, such as the chemicals in the stream, that have not been tagged, or described.
“In unsupervised learning, we look for patterns in the data, for example, clusters in the data and see what patterns emerge to be able to learn something new about the data set that we already have,” said Shaughnessy.
To test the model, the researchers gathered stream data collected from Shale Hills Critical Zone Observatory, a living laboratory established in 2007 near State College, Pennsylvania, where researchers gather data on important hydrological, ecological and geochemical processes in the watershed.
“It’s a site that has been operated and funded by the National Science Foundation for years,” said Brantley. “We’ve made a lot of measurements over the years there so we know a lot about that system and our set of math worked really great for that system, where we knew a lot about it.”
The team validated the algorithm using on data from two other sites around the country — East River, a large, mountainous watershed located near Gothic, Colorado, and Hubbard Brook, a series of nine small, forested watersheds located in the White Mountains of New Hampshire.
“It was a nice thing to be able to start the project at a Penn State place where we had a huge amount of data being collected, funded by NSF, and then move to other sites that had been funded and maintained by other people to show that it worked,” said Brantley. “It gave us different interpretations because the geology and other factors are different. But, the technique works and I think it’s going to be really useful technique that can help a lot of people understand stream chemistry.”
Currently, researchers are using the algorithm to investigate stream chemistry in the Marcellus Shale region, an area where fracking and mining may have impacted streams.
Brantley, who is also the director of the Earth and Environmental Systems Institute, and Shaughnessy worked with Xin Gu, assistant research professor in Earth and Environmental Systems Institute and Tao Wen, assistant professor of earth and environmental sciences, Syracuse University.
Share
Related Posts
- Multi-institutional team to use AI to evaluate social, behavioral science claims
- ICS Seed Grants to power projects that use AI, machine learning for common good
- A peek at living room decor suggests how decorations vary around the world
- Creepy computers or people partners? Working to make AI that enhances humanity