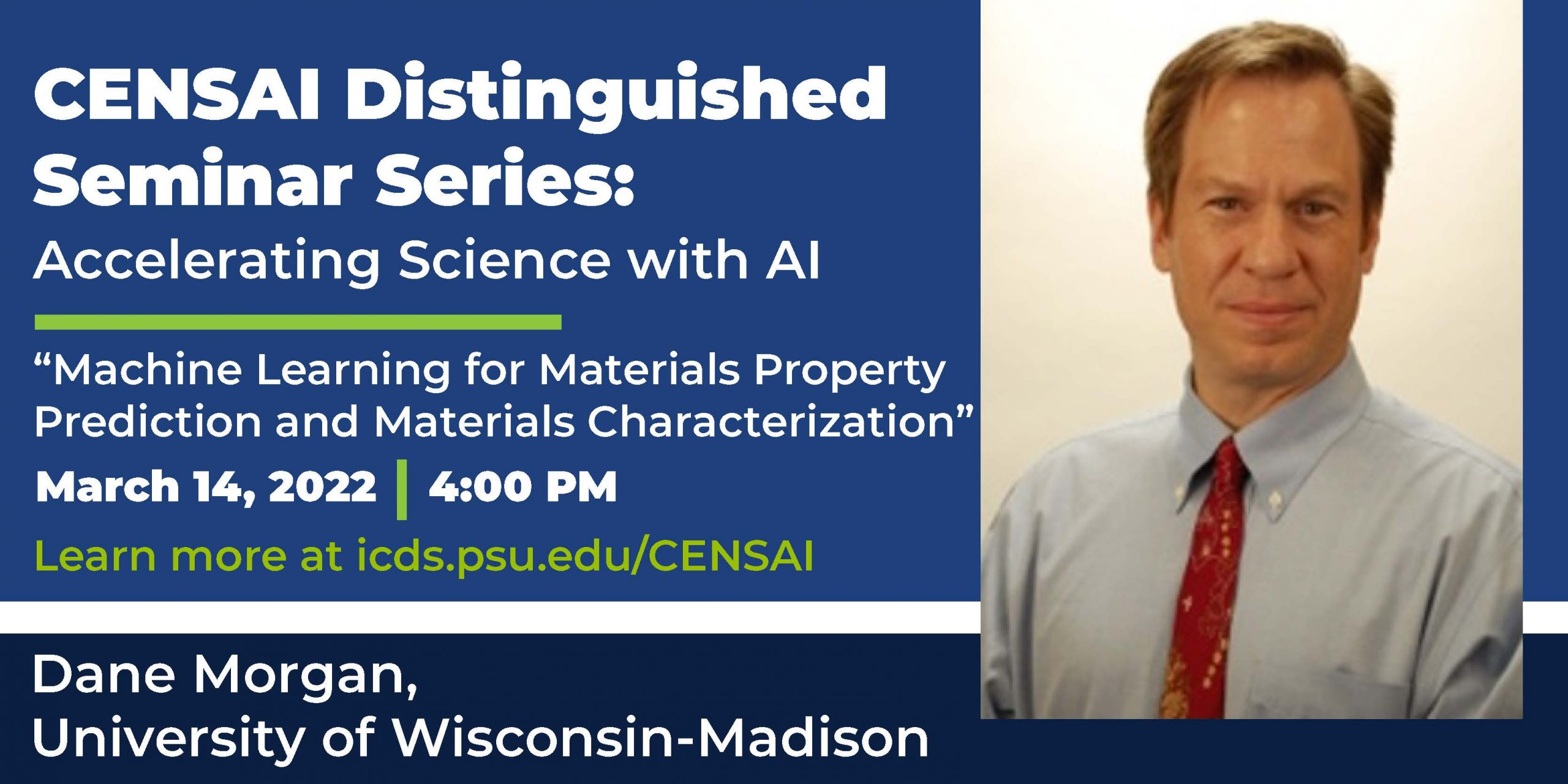
Distinguished AI Seminar: “Machine Learning for Materials Property Prediction and Materials Characterization”
Date: Monday, March 14
Time: 4:00 p.m.
Location: Online
Join ICDS and the Center for Artificial Intelligence Foundations and Scientific Applications (CENSAI) for the “Accelerating Science with AI” Distinguished Seminar Series. The Feb. 14 seminar will feature Dane Morgan, the Harvey D. Spangler Professor of Engineering, University of Wisconsin-Madison, with a talk titled “Machine Learning for Materials Property Prediction and Materials Characterization.”
Abstract:
Machine learning methods are playing an increasing role in materials research, from predicting properties to accelerating characterization to extracting data from text. In this talk I will give a brief overview of recent activities and opportunities for machine learning in materials. Then I will discuss recent efforts in my group in three areas. The first is assessing model domains and uncertainties in materials property prediction, where I will share assessments of common Bayesian (Gaussian process regression) and ensemble methods. We show that Gaussian process regression is better at determining model domain than ensembles, but that the ensembles error bars are more accurate when properly calibrated. I will also discuss our recent efforts to automate deep learning object detection approaches to find the location and geometry of different defect types in electron microscopy images of irradiated steels. We show that an accuracy comparable to human analysis can be achieved, suggesting a future where defect analysis is more standardized and orders of magnitude faster than today, but that training data requirements, transferability, and feature sizes are a challenge. Finally, I will discuss some successes and challenges fitting machine learning models to represent interactions between atoms, called machine learning potentials, which have the potential to dramatically accelerate a wide range of materials simulations. In all these examples I will try to illustrate where I think open challenges create opportunities for AI experts to help materials researchers integrate more effective machine learning approaches.
Bio:
Dane Morgan is a Professor in Materials Science and Engineering at the University of Wisconsin, Madison. His work combines thermostatistics, thermokinetics, and informatics analysis with atomic scale calculations to understand and predict materials properties. A major focus of Morgan’s work is energy applications, including fuel cells, batteries, and nuclear materials, but he also works in the areas of thermionic emitters and defect properties in semiconductors. He is the Harvey D. Spangler Professor of Engineering and a University of Wisconsin Vilas Scholar, received multiple teaching and research awards, and has published over 300 papers in materials science.