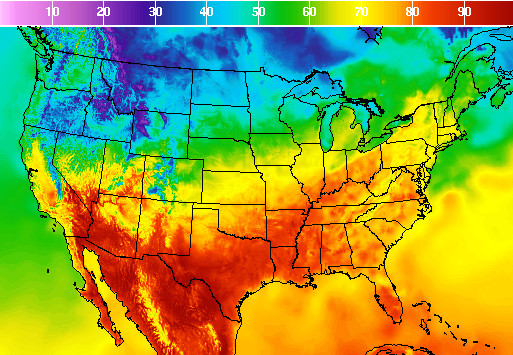
Artificial intelligence may be able to help weather forecasters focus their computational power on areas that experience more complex weather patterns -- and doing simpler and less computationally expensive estimations on other areas. Image: Wikimedia Commons
Focusing computational power for more accurate, efficient weather forecasts
Posted on August 23, 2019UNIVERSITY PARK, Pa. — They say if you don’t like the weather, just wait awhile. But how long you wait may depend on your location — the weather changes much faster and more violently in some geographic areas compared to others, which can mean that current weather prediction models may be slow and inefficient.
Now, Penn State researchers are using artificial intelligence to pinpoint those swift-changing weather areas to help meteorologists produce more accurate weather forecasts without wasting valuable computational power.
In a study, the researchers used an AI model based on natural selection to find areas of the continental United States where temperature changes are harder to predict and variable, so that computational resources can be focused there, rather than places were the weather is less apt to change. The resulting temperature prediction algorithm was equal to or better than the current model, but used less computational power.
According to Guido Cervone, professor of geography, meteorology and atmospheric science, Penn State and co-hire and associate director of the Institute for CyberScience, which provides Penn State researchers access to supercomputing resources, the study could lead to a solution to developing more accurate short-term forecasts, one of meteorology’s trickiest problems.
“Our methodology helps focus the available computational resources toward areas that are harder to predict, which in turn should help generate better short-term forecasts,” said Cervone. “Numerical weather prediction is one of the most computationally demanding problems, and its use for society is far-reaching.”
Weiming Hu, a doctoral student in geography, said that the current weather maps are divided up into a simple mesh of about 200,000 grid points in the United States. When weather forecasters use computers to analyze weather patterns in those areas, the computational power is spread equally among those grid points, which each represent about 11 kilometers, or 7 miles, in diameter. While that might sound like common sense, Hu said the map does not reflect the computational reality of weather prediction. Topography, elevation, the proximity of water and a myriad of other factors can disrupt weather patterns, making certain areas much more difficult to predict.
“If you think about Iowa, let’s say, it rarely experiences huge changes in the weather regimes across dozens of kilometers, compared to some other places, because the topography is relatively simple and you can use some very easy interpolation — or estimates — to give you some good ideas about, in this case, what the temperature will be in the future,” said Hu. “But, in the Rocky Mountains, you can go from the plains to the peak of a mountain in just a few kilometers and that changes things dramatically when you’re trying to predict weather regimes. What we want to address is how can we figure out what are the more important or more interesting areas where we need either to have a higher resolution or a more accurate weather prediction for that specific region.”
The researchers, who published their findings in the Journal of Computers and Geosciences, currently online, used genetic algorithms to help create a more flexible mesh to focus computational analysis on grids with complex, rapidly changing weather patterns. The mesh in other areas of the country, where the weather is steadier, can be expanded.
Hu said genetic algorithm programs are a machine learning model that is loosely based on biological evolution. In biological evolution, only a few individuals will survive in a certain environment out of the thousands that attempted to live there. Similarly, in genetic programming, hundreds or thousands of potential solutions will be tested to superior ones, such as, in this case, locations in need of a finer mesh grid.
Hu added that genetic algorithms are designed to offer good solutions, rather than perfect ones.
“Genetic algorithms do not guarantee the best solution, but they do guarantee finding better solutions faster,” said Hu. “In a case like predicting temperature changes you might not care about finding the ultimate solution because it might be the difference between 29.56 degrees and 29.55 degrees. That’s probably not going to matter for the regular person.”
While the researchers’ study looked specifically at temperature change, Hu said that in the future the model could be tested on other weather conditions, such as precipitation and cloud cover.
The National Science Foundation supported this work.
Share
Related Posts
- Multi-institutional team to use AI to evaluate social, behavioral science claims
- Predictability limit: Scientists find bounds of weather forecasting
- ICS Seed Grants to power projects that use AI, machine learning for common good
- A peek at living room decor suggests how decorations vary around the world