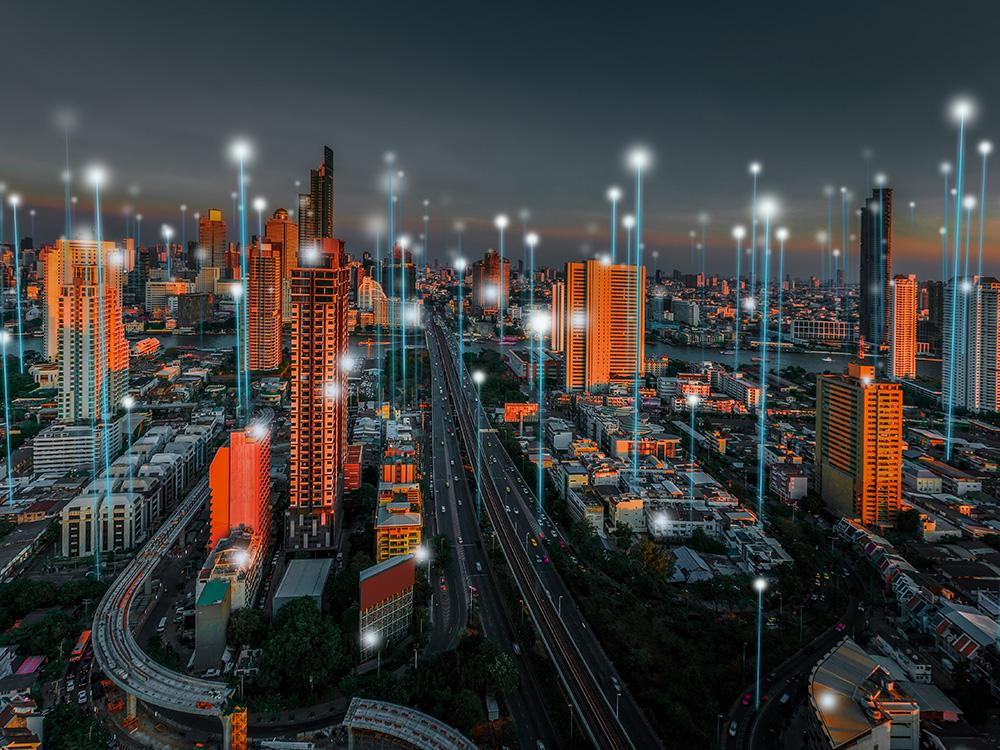
A Penn State-led research team developed a scalable model to control large groups of buildings and their energy demand in realistic operational settings. Credit: Urupong/iStock. All Rights Reserved.
Scalable model makes for more efficient, smarter grid use, researchers report
Posted on November 30, 2022UNIVERSITY PARK, Pa. — Buildings consume roughly 75% of the electricity used in the United States, and, without significant changes, the number is expected to rise in the coming decades. An answer potentially exists in renewable resources, but they are plagued with the uncertainty of when the sun might shine, when the wind might blow and how best to plug into the grid. There may be a method to control the madness, though, according to Greg Pavlak, assistant professor of architectural engineering at Penn State.
Computational models to optimize how a building uses renewable energy exist but tend to falter as the number of buildings they need to optimize increases, Pavlak said. Pavlak and his collaborators developed a scalable model specifically to control large groups of buildings and their energy demand in realistic operational settings. They published their approach in the Journal of Energy Storage.
“Our approach allows us to control large portfolios of buildings in an intelligent way, using renewable energy and thermal storage,” Pavlak said. “To make those control decisions, we need to account for uncertainty. There are a lot of unknowns: what’s the weather, what are people doing, what is the current energy cost and more. Our model takes all of those uncertainties into account to develop the best plan.”
The model applies predictive stochastic optimization, meaning the variables are uncertain but have likely distributions. For example, if there is an 80% chance of rain tomorrow, the model can reasonably predict that there will be less sunlight beaming to the grid, but that it will also be cooler so less electricity may be needed to power air conditioner across a suite of buildings.
“We developed a supervisory controller reliant on solving a stochastic planning problem followed by solving a sequence of real-time operational problems,” Pavlak said. “Crucial to the practical implementation of this framework, we propose a parallel implementation of a smoothing-based variance-reduced gradient method that displays the optimal rate and near-optimal sample complexity that can scale with a number of scenarios.”
In other words, there are two key functions to the framework. The first is creating a day-ahead plan for building operations based on various real-world scenarios, such as weather or building occupancy, estimates for which are pulled from recent history and forecasts. The second is real-time optimization to adjust the plan according to actual conditions. The optimized solution is combined with the day-ahead predictions, and, together, the framework can efficiently solve the demand question across scales, according to Pavlak.
The team simulated two case studies to compare their solution with traditional approaches to test efficiency, scalability and outcome with regard to known information. They used two past dates with known variables for the comparison. On average, their solution provided savings of roughly $50 per day and up to $150 per day.
The proposed solution algorithm is more scalable than current state-of-the-art solvers in terms of computer memory use and processing time, according to Pavlak.
“This means we can now solve real-world decision-making problems that involve uncertainty across many buildings, systems and devices,” Pavlak said.
The new model is the starting point of possibility, Pavlak said, especially as new energy market structures that more directly incorporate flexible resources evolve.
“We’re beginning to develop simpler models that scale up to larger numbers, resulting in more economic and efficient control mechanisms,” Pavlak said. “We’re preparing the decision-making tools that will be needed for those new energy markets in the future.”
Other contributors include Min Gyung Yu, who graduated with a doctorate in architectural engineering from Penn State in 2022 and is now a control system engineer at the Pacific Northwest National Laboratory; and Uday V. Shanbhag, the Gary and Sheila Bello Chair Professor in the Harold and Inge Marcus Department of Industrial and Manufacturing Engineering at Penn State.
Pavlak is also a co-funded faculty member with the Penn State Institutes of Energy and the Environment, which supported this work. The computations for this research were performed on the Penn State Institute for Computational and Data Sciences’ Roar supercomputer.