The Institute for AI-Enabled Materials, Discovery, Design, and Synthesis (AIMS) hosts events to build collaborations among participating AIMS institutions.
Upcoming Events
No events are scheduled at this time.
Past Events
September 12, 2022: “Autonomous Materials Research and Discovery at NIST”
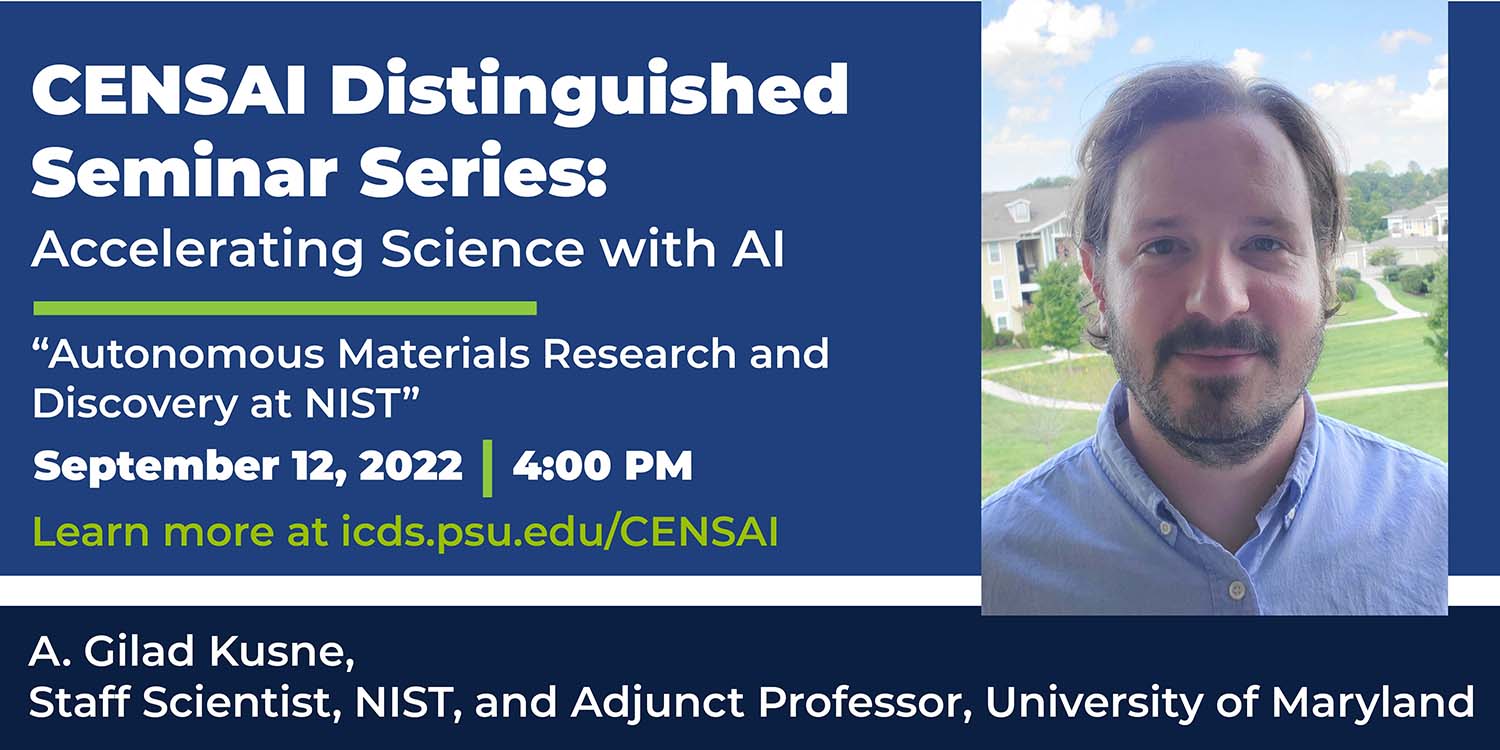
Abstract: The last few decades have seen significant advancements in materials research tools, allowing scientists to rapidly synthesize and characterize large numbers of samples – a major step toward high-throughput materials discovery. Autonomous research systems take the next step, placing synthesis and characterization under control of machine learning. For such systems, machine learning controls experiment design, execution, and analysis, thus accelerating knowledge capture while also reducing the burden on experts. Furthermore, physical knowledge can be built into the machine learning, reducing the expertise needed by users, with the promise of eventually democratizing science. In this talk I will discuss autonomous systems being developed at NIST with a particular focus on autonomous control over user facility measurement systems for materials characterization, exploration and discovery.
Bio: A. Gilad Kusne received his B.S., M.S., and Ph.D. degrees from Carnegie Mellon University. He is a Staff Scientist with the National Institute of Standards and Technology (NIST), Gaithersburg, Maryland, an adjunct professor with the University of Maryland, and a Fellow of the American Physical Society. His research is part of the White House’s Materials Genome Initiative at NIST, a project which aims to accelerate the discovery and optimization of advanced materials. He leads the machine learning team of an international, cross-disciplinary effort building autonomous research systems, with the goal of advancing solid state, soft, and biological materials. For these systems, machine learning performs experiment design, execution (in the lab and in silico), and analysis. For his work, he has been awarded the NIST Bronze Award (highest NIST award) and APS Fellow. He is also the lead founder and organizer of the annual Machine Learning for Materials Research Bootcamp and Workshop—educating next generation and mid-career material scientists in machine learning.
March 14, 2022: “Machine Learning for Materials Property Prediction and Materials Characterization”
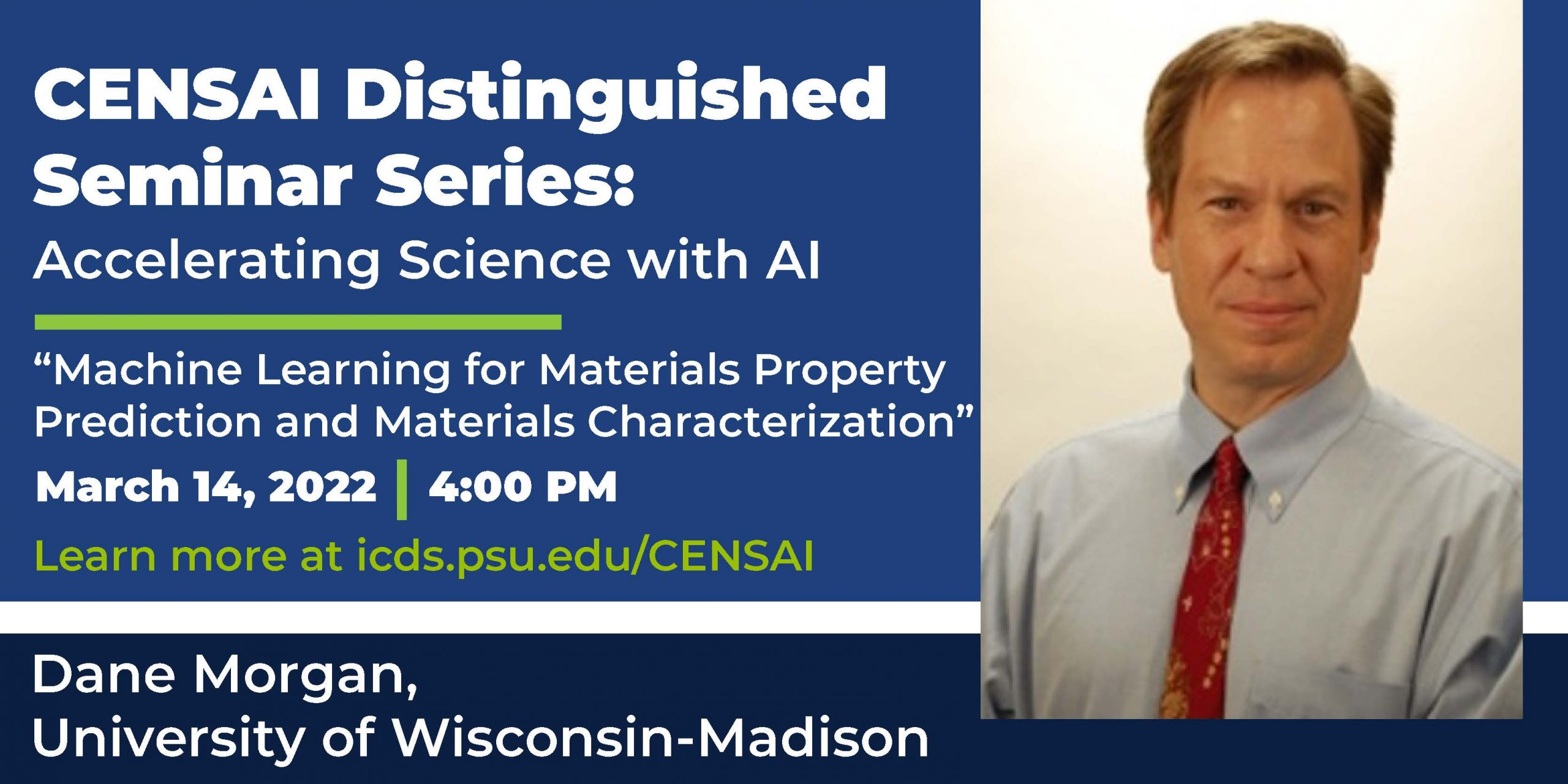
Abstract: Machine learning methods are playing an increasing role in materials research, from predicting properties to accelerating characterization to extracting data from text. In this talk I will give a brief overview of recent activities and opportunities for machine learning in materials. Then I will discuss recent efforts in my group in three areas. The first is assessing model domains and uncertainties in materials property prediction, where I will share assessments of common Bayesian (Gaussian process regression) and ensemble methods. We show that Gaussian process regression is better at determining model domain than ensembles, but that the ensembles error bars are more accurate when properly calibrated. I will also discuss our recent efforts to automate deep learning object detection approaches to find the location and geometry of different defect types in electron microscopy images of irradiated steels. We show that an accuracy comparable to human analysis can be achieved, suggesting a future where defect analysis is more standardized and orders of magnitude faster than today, but that training data requirements, transferability, and feature sizes are a challenge. Finally, I will discuss some successes and challenges fitting machine learning models to represent interactions between atoms, called machine learning potentials, which have the potential to dramatically accelerate a wide range of materials simulations. In all these examples I will try to illustrate where I think open challenges create opportunities for AI experts to help materials researchers integrate more effective machine learning approaches.
Bio: Dane Morgan is a Professor in Materials Science and Engineering at the University of Wisconsin, Madison. His work combines thermostatistics, thermokinetics, and informatics analysis with atomic scale calculations to understand and predict materials properties. A major focus of Morgan’s work is energy applications, including fuel cells, batteries, and nuclear materials, but he also works in the areas of thermionic emitters and defect properties in semiconductors. He is the Harvey D. Spangler Professor of Engineering and a University of Wisconsin Vilas Scholar, received multiple teaching and research awards, and has published over 300 papers in materials science.
November 15, 2021: “Digital transformation in biologics drug discovery: challenges and opportunities”
Abstract: More, better, faster… and now smarter. Sanofi’s Large Molecule Research (LMR) (i.e. biologics drug discovery and development organization) is on its journey towards the promise of advanced computation. At Sanofi LMR we aim to turn innovative science into novel biologic candidates to improve the health of patients. We need to provide a robust engine to discover and deliver innovative biologics to conquer complex diseases via multi-targeting, smart biologics, and intelligent drug design. In this talk, I will provide an introduction to the challenges of discovering and developing large-molecule drugs (biologics) focusing on molecule design and describe the application landscape of AI/ML in this domain. I will provide an overview of our efforts to intensify the use of data and computation in all aspects of biologics discovery and optimization; and to transform to next-gen processes driven by ML/AI innovations.
Speaker Bio: Maria Wendt is VP Head of Biologics Research US and Global Head of Digital Biologics Platform, Large Molecule Research at Sanofi, based in Framingham and Cambridge, MA. She has spent the last 20 years at the interface of computation and biology. At Sanofi, she oversees the internal and collaborative biologics discovery programs for multiple therapeutic areas in the US, and the platform innovation portfolio including use of ML/AI. Prior to Sanofi, she was Head of Science at Genedata AG in Basel, Switzerland where she led the creation of Genedata Biologics and Genedata Bioprocess, foundational enterprise informatics platforms in use at most top global biopharma today. In the early part of her career, she focused on -omics Big Data and was one of the leaders of the EU Framework 6 InnoMed Predictive Toxicology consortium. She earned her Ph.D. at Iowa State University in Chemical Engineering.
October 18, 2021: “Computational Sustainability: Computing for a Better World and a Sustainable Future”
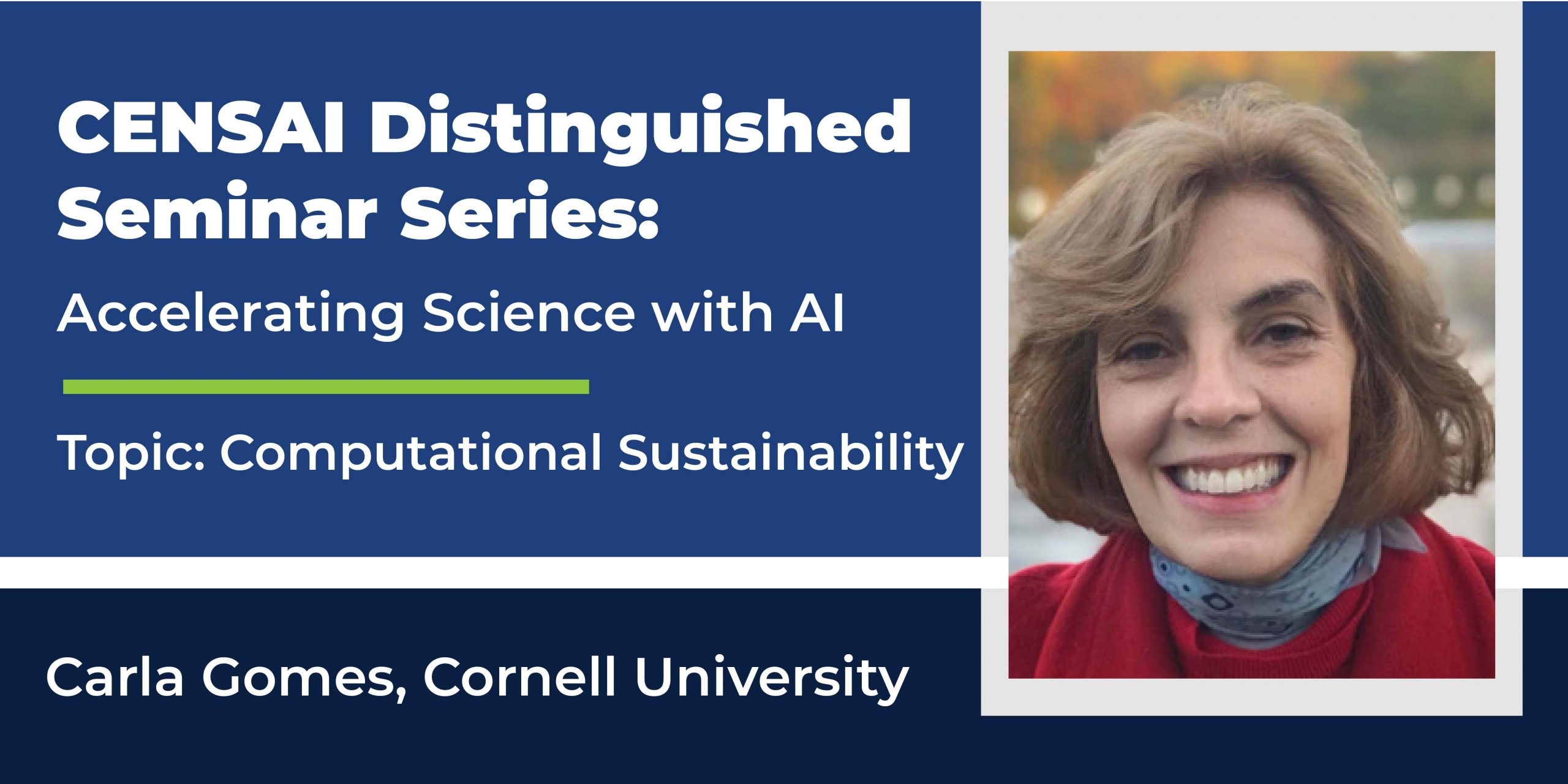
Abstract: Artificial Intelligence (AI) is a rapidly advancing field. Novel machine learning methods combined with reasoning and search techniques have led us to reach new milestones: from computer vision, machine translation, and Go world-champion level play, to self-driving cars. These ever-expanding AI capabilities open up new exciting avenues for advances in new domains. I will discuss our AI research for advancing scientific discovery for a sustainable future. In particular, I will talk about our research in a new interdisciplinary field, Computational Sustainability, which has the overarching goal of developing computational models and methods to help manage the balance between environmental, economic, and societal needs for a sustainable future. I will provide examples of computational sustainability problems, ranging from biodiversity and wildlife conservation, to multi-criteria strategic planning of hydropower dams in the Amazon basin and materials discovery for renewable energy materials. I will also highlight cross-cutting computational themes and challenges for AI at the intersection of constraint reasoning, optimization, machine learning, multi-agent reasoning, and citizen science.
About the Speaker: Carla Gomes is the Ronald C. and Antonia V. Nielsen Professor of Computing and Information Science and the director of the Institute for Computational Sustainability at Cornell University. Gomes received a Ph.D. in computer science in the area of artificial intelligence from the University of Edinburgh. Her research area is Artificial Intelligence with a focus on large-scale reasoning, optimization, and learning. Recently, Gomes has become deeply immersed in research on scientific discovery for sustainability and more generally research in the new field of Computational Sustainability. Computational Sustainability aims to develop computational methods to help solve some of the key challenges concerning environmental, economic, and societal issues in order to help put us on a path towards a sustainable future. From 2007-2013 Gomes led an NSF Expeditions-in-Computing in Computational Sustainability that nucleated the new field of Computational Sustainability. Gomes is currently the lead PI of a new NSF Expeditions-in-Computing that established CompSustNet, a large-scale national and international research network, to further expand the field and Computational Sustainability. Gomes has (co-)authored over 150 publications, which have appeared in venues spanning Nature, Science, and a variety of conferences and journals in AI and Computer Science, including five best paper awards. Her research group has been supported by over $50M in basic research funds. Gomes is a Fellow of the Association for the Advancement of Artificial Intelligence (AAAI), a Fellow of the Association for Computing Machinery (ACM), and a Fellow of American Association for the Advancement of Science (AAAS).
April 9, 2021: NSF Workshop on AI & Materials
Join AIMS for the “NSF Workshop on Accelerating Materials Discovery, Design, and Synthesis: A Grand Challenge for Artificial Intelligence” from 10:00 a.m. to 4:00 p.m. ET on Friday, April 9. One of the main goals of the workshop is to identify opportunities for synergistic advances in AI (including machine learning, automated planning and optimization of experiments, multi-objective optimization, human-AI interaction, and more) and material science and engineering.
The workshop will include a keynote and three panel discussions, featuring researchers from 15 universities or government organizations.
March 26, 2021: “Bridging the Gap Between Literature Data Extraction and Domain Specific Materials Informatics”
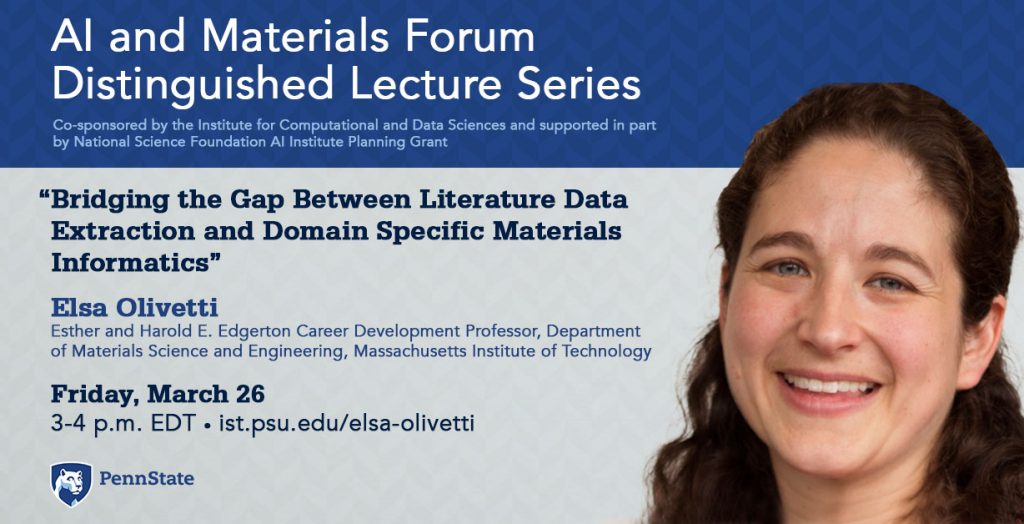
Abstract: Data has become a fundamental ingredient for accelerating and optimizing materials design and synthesis. Advances in applying natural language processing (NLP) to material science text has greatly increased the size and acquisition speed of materials science data from the published literature. This presentation will describe work to extract information from peer reviewed academic literature across a range of materials. Applying NLP pipelines to these types of materials science systems can be challenging due to the general schema and the noisiness of automatically extraction data. Dr. Olivetti will present data engineering techniques and discuss an optimal balance between automatic and manual data extraction.
About the Speaker: Elsa Olivetti is the Esther and Harold E. Edgerton Career Development Professor in the Department of Materials Science and Engineering (DMSE) at the Massachusetts Institute of Technology. Her research focuses on improving the environmental and economic sustainability of materials in the context of rapid-expanding global demand. Dr. Olivetti received her B.S. degree in Engineering Science from the University of Virginia and her Ph.D. in Materials Science Engineering from MIT.
March 5, 2021: “Predicting Materials Properties at Scale with Machine Learning”
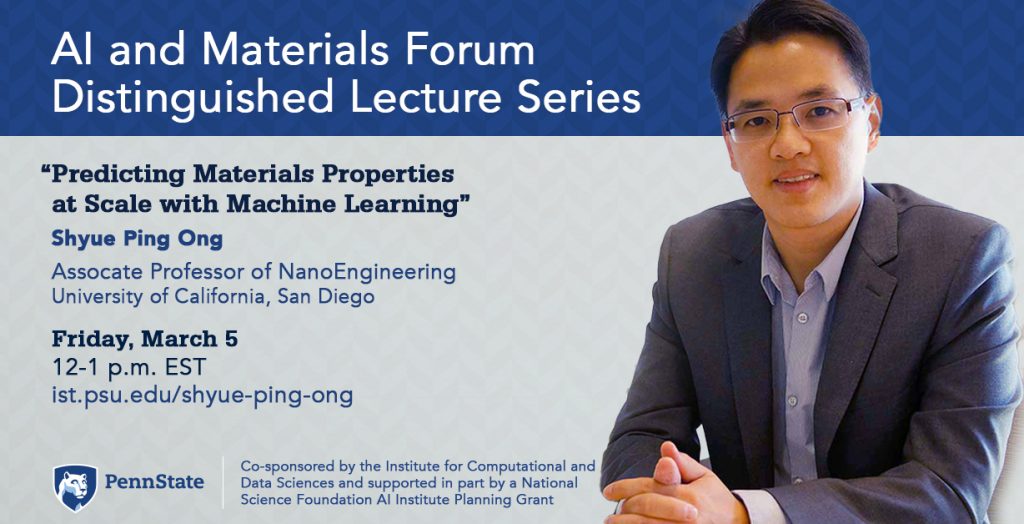
Abstract: Machine learning (ML) models have demonstrated human, or even superhuman, performance in many tasks, from playing traditional board games to image classification. In this webinar, I will discuss how ML is poised to have a similar transformative impact in materials science. Applied on large data sets, ML techniques can be used to discover novel technological materials, to model complex systems at an accuracy beyond the reach of traditional computational techniques, and to enhance the accuracy and speed of interpreting characterization data. A key focus of this talk will be on the heterogeneity and scarcity of materials data, the challenges these characteristics present for ML, and the potential approaches to overcome them.
About the Speaker: Dr. Shyue Ping Ong is an Associate Professor of NanoEngineering at the University of California, San Diego. He obtained his Ph.D. from the Massachusetts Institute of Technology in 2011. His group, the Materials Virtual Lab, is dedicated to the interdisciplinary application of machine learning and first principles computations to accelerate materials design. He is a key developer of the Materials Project and the globally used Python Materials Genomics (pymatgen) materials library. Dr. Ong is also a recipient of the U.S. Department of Energy Early Career Research Program and the Office of Naval Research Young Investigator Program awards.
February 26, 2021: “Physical Discovery by Machine Learning: from Symmetries and Chemical Reactions to Generative and Causal Models”
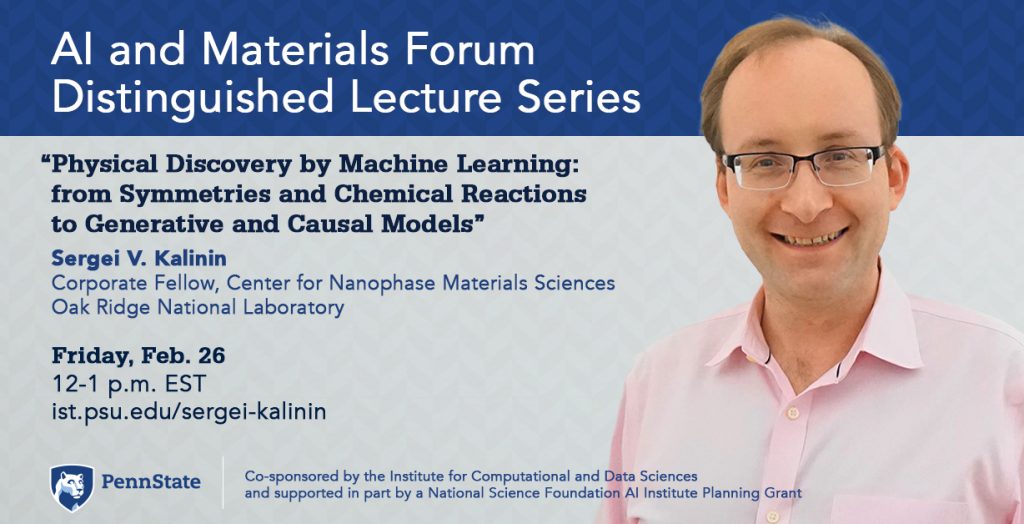
Abstract: Machine learning has emerged as a powerful tool for the analysis of mesoscopic and atomically resolved images and spectroscopy in electron and scanning probe microscopy. The applications ranging from feature extraction to information compression and elucidation of relevant order parameters to inversion of imaging data to reconstruct structural models have been demonstrated. In this presentation, I will discuss several applications of autoencoders and variational autoencoders for the analysis of image and spectral data in STEM and SPM. The special emphasis is made on the rotationally invariant variational autoencoders that allow to disentangle rotational degrees of freedom from other latent variables in imaging and spectral data. The analysis of the latent space of autoencoders further allows establishing physically relevant transformation mechanisms. Extension of encoder approach towards establishing structure-property relationships will be illustrated on the example of ferroelectric domain walls and plasmonic structures. I will further illustrate the applications of the Bayesian inference methods towards inferring the mesoscopic and atomistic physics of materials in terms of continuous and atomistic generative models, and illustrate the pathways towards incorporation of physical models as priors within Bayesian optimization towards effective sampling of experimental parameter spaces. Ultimately, we seek to answer the causal questions such as whether frozen atomic disorder drives the emergence of the local structural distortions or average shift of the Fermi level induces structural reconstruction that in turn drive cation distribution, whether the nucleation spot of phase transition can be predicted based on observations before the transition, and what is the driving forces controlling the emergence of unique functionalities in quantum materials.
This research is supported by the by the U.S. Department of Energy, Basic Energy Sciences, Materials Sciences and Engineering Division and the Center for Nanophase Materials Sciences, which is sponsored at Oak Ridge National Laboratory by the Scientific User Facilities Division, BES DOE.
About the Speaker: Sergei V. Kalinin is a corporate fellow and a group leader at the Center for Nanophase Materials Sciences at Oak Ridge National Laboratory. He received his MS degree from Moscow State University in 1998 and Ph.D. from the University of Pennsylvania (with Dawn Bonnell) in 2002. His research presently focuses on the applications of big data and artificial intelligence methods in atomically resolved imaging by scanning transmission electron microscopy and scanning probes for applications including physics discovery and atomic fabrication, as well as mesoscopic studies of electrochemical, ferroelectric, and transport phenomena via scanning probe microscopy.
Sergei has co-authored >650 publications, with a total citation of >33,000 and an h-index of >94. He is a fellow of MRS, APS, IoP, IEEE, Foresight Institute, and AVS; a recipient of the Blavatnik Award for Physical Sciences (2018), RMS medal for Scanning Probe Microscopy (2015), Presidential Early Career Award for Scientists and Engineers (PECASE) (2009); Burton medal of Microscopy Society of America (2010); 4 R&D100 Awards (2008, 2010, 2016, and 2018); and a number of other distinctions.
February 12, 2021: “Artificial Intelligence for materials design: advances and remaining challenges”
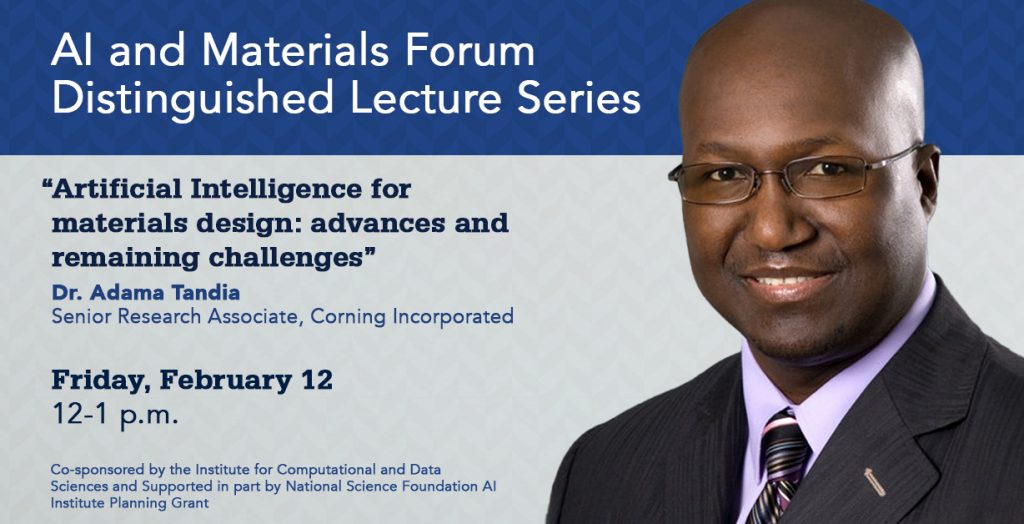
Abstract:
Use of artificial intelligence with materials data sets has significantly improved the speed of discovery of new materials with improved attributes. In the field of glass and polymer design, combinations of different regression methods, such as genetic algorithm, Gaussian processes, and neural networks, and data generation methods, such as molecular dynamics and density functional theory, have led to robust design methods and near defect free manufacturing processes. Yet, despite the many successes, many serious challenges for faster and more robust algorithms remain before the design and manufacture of materials with optimal characteristics.
Bio:
Dr. Adama Tandia received his Ph.D. in Applied Mathematics/Applied Physics from Paul Sabatier University (France) in 1998. He worked at the Department of Applied Mathematics at Northwestern University before joining the Department of Modeling & Simulation at Corning Incorporated since 2000. Tandia is a recognized subject matter expert in applications of molecular modeling and machine learning for materials design and process optimization. In 2008, Tandia introduced the use of machine learning at Corning and led the uphill charge for its widespread across the company.
January 29, 2021: “Domain-specific Considerations in Machine Learning for Materials Design”
Abstract: Machine learning (ML) offers a promising path to significantly accelerating the development of new materials. At Citrine, we have found that a materials-tailored approach (rather than domain-agnostic ML) is crucial to success. For example, uncertainty quantification (UQ) can help prioritize candidate materials within vast design spaces, and physics-based simulations can provide valuable training data for transfer learning when experiments are scarce. In this talk, I will outline several examples of materials-tailored ML method development, and also highlight promising areas for future research.
About the Speaker: Dr. Bryce Meredig is cofounder and Chief Science Officer of Citrine Informatics, a materials informatics platform company, where he leads the External Research Department (ERD). ERD conducts non-proprietary, publishable research with collaborators in academia, government, and industry. Dr. Meredig’s research interests include the development and validation of physics-informed machine learning methods specific to applications in materials science and chemistry; integration of physics-based simulations with machine learning; and data infrastructure for materials science. Dr. Meredig earned his PhD from Northwestern University and BAS and MBA from Stanford University.