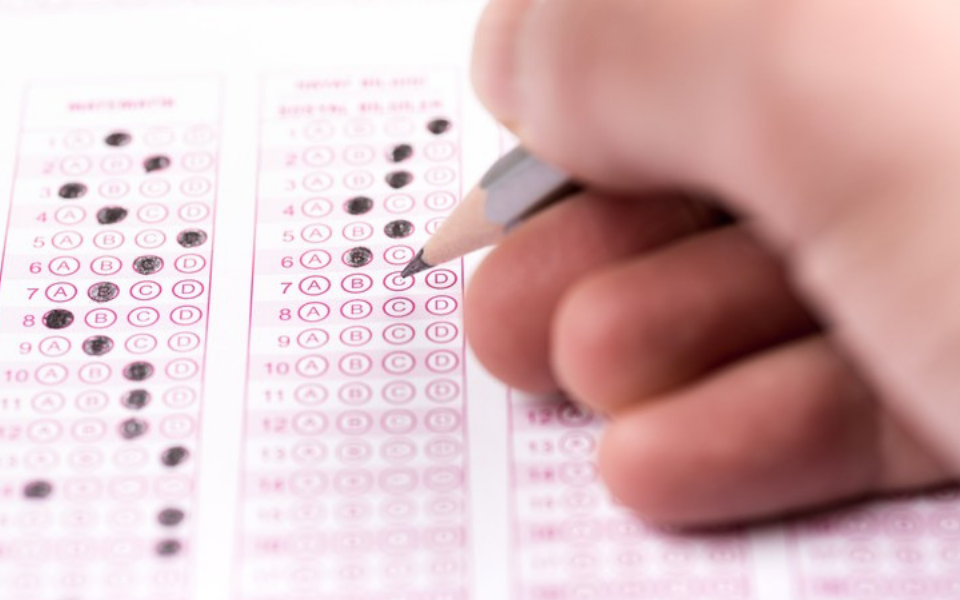
Putting answers to the test
Posted on September 24, 2018UNIVERSITY PARK, Pa. — Teachers, rejoice. A team of Penn State researchers has developed a way to make multiple-choice exam questions easier for instructors to create, yet more intellectually challenging for test-takers.
Utilizing machine-learning ranking models, the researchers have proposed a method that automatically generates “distractors,” which are the alternative options used to distract students from the correct answer in a multiple-choice question.
“Among all methods for creating good multiple-choice questions, finding reasonable distractors is crucial and usually the most time consuming,” the researchers wrote in their paper.
Looking at a multiple-choice question’s stem (the question sentence) and its key (the correct answer), the researchers created an algorithm that automatically generates plausible false answers. They defined a good distractor as being semantically related to the key and the stem, as well as being grammatically correct.
Chen Liang, a member of the research team who earned his doctorate from Penn State’s College of Information Sciences and Technology in May, said that his team’s proposed method stands apart from existing models of creating distractor answers, such as those based on ontology and similarity. In these existing methods, the distractors are selected from a distractor set based on a weighted combination of similarities, such as phonetic or syntactic parallels, often using rules of thumb by the exam creator.
“Our proposed methods differ from [existing] methods in that we use machine and deep learning to learn the ‘unknown’ relation between the question and ‘good’ distractors from existing multiple choice questions,” he said. “The idea is to learn from a large amount of real multiple-choice questions instead of using heuristics as existing methods do.”
“Through empirical experiments, we are able to show that proposed learning-based models significantly outperform existing distractor generation models,” he added.
The researchers hope that their method will provide teachers with a tool to create stimulating multiple-choice questions. The goal is to incorporate the method to generate multiple-choice questions as exercises for book chapters in a computer-facilitated book-creation system that Liang helped to develop in 2016, and that the research will continue to learn how to generate questions given a large piece of text, such as a paragraph or chapter.
“Our collaborators in Teaching and Learning with Technology (TLT) are developing a question-building tool for teachers that utilizes our algorithms,” said C. Lee Giles, David Reese Professor of Information Sciences and Technology and a member of the research team. “The intent is for the tool to be incorporated into Penn State’s systems that will allow professors to create multiple-choice questions. We believe this will help teachers readily design better multiple-choice questions.”
“We are really excited to see what happens when we build a tool around these algorithms, and put the tool in the hands of teachers,” added Bart Pursel, assistant director of TLT Innovation. “While the current results comparing our method to other methods of distractor generation are promising, we really won’t know how good these distractors are until teachers use this technique in an authentic way, creating multiple-choice questions that students answer as part of formative or summative assessments.”
The team’s paper, “Distractor Generation for Multiple Choice Questions Using Learning to Rank,” was presented at the 13th Workshop on Innovative Use of NLP for Building Educational Applications at the 16th Annual Conference of the North American Chapter of the Association for Computational Linguistics: Human Language Technologies. The work was funded primarily by the Center for Online Innovation in Learning at Penn State.
Other researchers on the project include Neisarg Dave, graduate student in the College of IST; graduate student Xiao Yang and professor Rebecca Passonneau from the School of Electrical Engineering and Computer Science; and Drew Wham, data scientist at Penn State’s Teaching and Learning with Technology.
“Our goal is to explore more ways artificial intelligence and machine learning can help improve and assist education,” Giles said. “In many ways this is just the beginning of what can be done.”