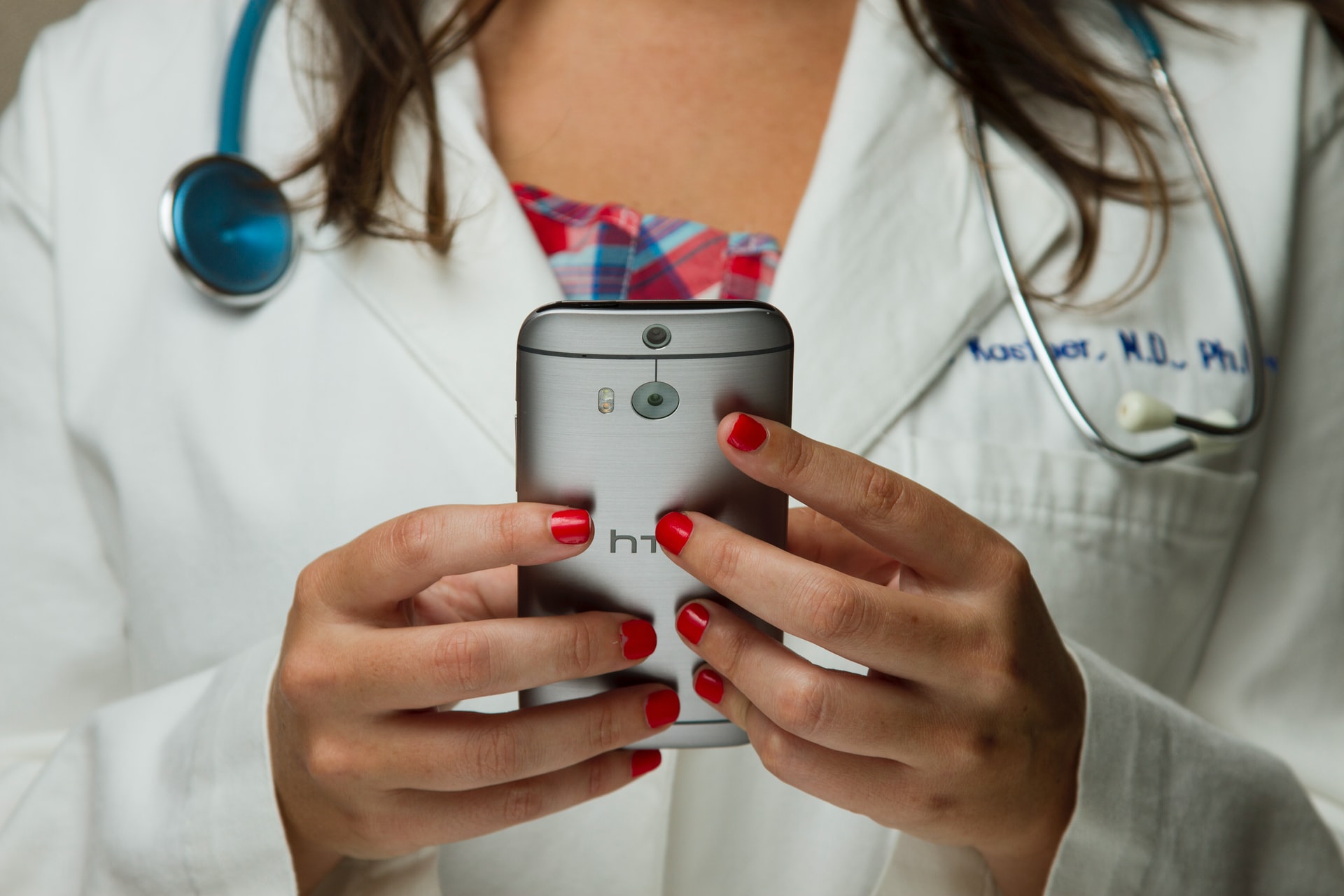
Interactivity improves transparency, deepens trust of AI symptom checkers
Posted on May 4, 2022NEW ORLEANS — Just as good doctors establish rapport with their patients, health chatbots programmed for conversational back-and-forth with patients may earn more trust from users and help them better understand their health assessments, according to Penn State researchers.
In a study, researchers said that people were significantly more likely to find an online symptom checker that responded to patients’ questions about anxiety through interactive dialogues to be more transparent and more trustworthy compared to a chatbot that provided a static webpage with explanations and one that provided no explanations at all. The interactive chatbot users also learned more about how their anxiety level was determined, the researchers added.
Because health advice can be literally a matter of life and death, artificially intelligent (AI) systems should be designed to be more transparent to inspire trust, said S. Shyam Sundar, James P. Jimirro Professor of Media Effects in the Donald P. Bellisario College of Communications and co-director of the Media Effects Research Laboratory at Penn State.
“There is a greater need for transparency, especially when people interact with AI systems or automated systems that deal with your health,” said Sundar, who also is an affiliate of Penn State’s Institute for Computational and Data Sciences (ICDS). “That transparency comes in the form of the system explaining itself. When a human doctor tells you that you have a particular condition because the symptoms correspond to a particular disease, you appreciate their effort to explain. How can we get AI systems to do the same and explain their decisions?”
Currently, online symptom checkers mimic human-like communication when diagnosing health conditions, yet the explanations of the assessment that they provide are often missing or provided separately as a “wall of text.” The findings of this study suggest that when explaining AI health systems, developers should leverage the power of conversations and make the explanations more conversational by providing back-and-forth interactive dialogue with the patient.
“An interactive dialogue gives you what you want to know and what you need to know, and it is all dictated by you, so you’re in the driver’s seat,” said Sundar, who also serves as director for Penn State’s Center for Socially Responsible AI. “That, then, makes the explanation from the system look more like it is catering to your needs. Users tend to have higher trust than when the system offers a pre-scripted text, almost like legal disclosure, which doesn’t inspire that much trust.”
Besides trusting the interactive systems more, participants also reported that they felt that they understood more about anxiety compared to people who used the static chatbot. In addition to this perceived knowledge, study participants who used the interactive chatbot also demonstrated more objective knowledge by correctly answering a significantly higher number of quiz questions about how the system makes health assessments, compared to static chatbot users, according to Yuan Sun, first author of the paper and doctoral candidate in mass communications at Penn State.
“Objective understanding is another important issue,” Sun said. “AI systems should empower patients to gain real knowledge of the systems so that they can make informed decisions.”
Avoiding the Black Box
According to the researchers, people may instinctively not trust AI doctors because they cannot tell how the machine is making the decision, often called the “black-box” problem of AI.
“One of the issues with AI algorithms is that they are unexplainable — people don’t know how they work,” said Sun. “When an algorithm is making a decision, for example, on a disease, it’s using a complicated process with things like rules-based logic and decision trees. What we are trying to communicate are the parts that people do understand, to make the process seem less complex and more trustworthy.”
She added that establishing this back-and-forth between the machine and the patient is also a more intuitive way to explain complicated information to patients.
“Even in a classroom, if a student asks the teacher to explain something, the teacher probably just won’t pull all of the information off of a page or a few pages and say, ‘Here you go,’” said Sun. “A more effective way would be for the teacher to answer the first question, then ask the students if they had another question, and so on. And then move on, once all of the students’ questions are answered.”
On the other hand, when users read all the information on a single page, they may feel overwhelmed and therefore choose to bypass the explanations.
Sun said that as telemedicine and online health care becomes more prevalent, health care organizations need to make sure that patients trust their AI systems and remove some of this natural hesitancy to AI.
“The main motivation of a study like this is to apply our knowledge from a social science perspective to really help improve trust because there is some hesitancy toward interacting with AIs, which suffer from this black box issue,” said Sun. “We want to address this chatbot hesitancy of patients who may stay away from AI health advice because they don’t know what’s happening and they don’t understand how these machines could, for example, diagnose their medical conditions.”
The researchers recruited 152 participants for the online experiment. The participants were assigned to one of three versions of a text-based chatbot designed to assess generalized anxiety disorder. The versions included an interactive chatbot that responded to the participants’ questions on anxiety, a static version that explained anxiety in general, and a control condition version that offered no explanation.
Participants were asked to rate their perceptions about their experience with the symptom checker, including ratings on the transparency of and their trust in the system.
Perceived understanding was measured through a survey that asked participants to rate their confidence in understanding how algorithms could diagnose anxiety. The researchers asked participants to answer five questions on how the symptom checker determined anxiety levels to measure objective knowledge.
The researchers presented their findings today (May 3) at the ACM Conference on Human Factors in Computing Systems (CHI’22), and reported them in its proceedings, the premier publication for research on human-computer interaction.
Share
Related Posts
- ICS Seed Grants to power projects that use AI, machine learning for common good
- Multi-institutional team to use AI to evaluate social, behavioral science claims
- Researcher to discuss threats to machine learning and AI applications
- A peek at living room decor suggests how decorations vary around the world