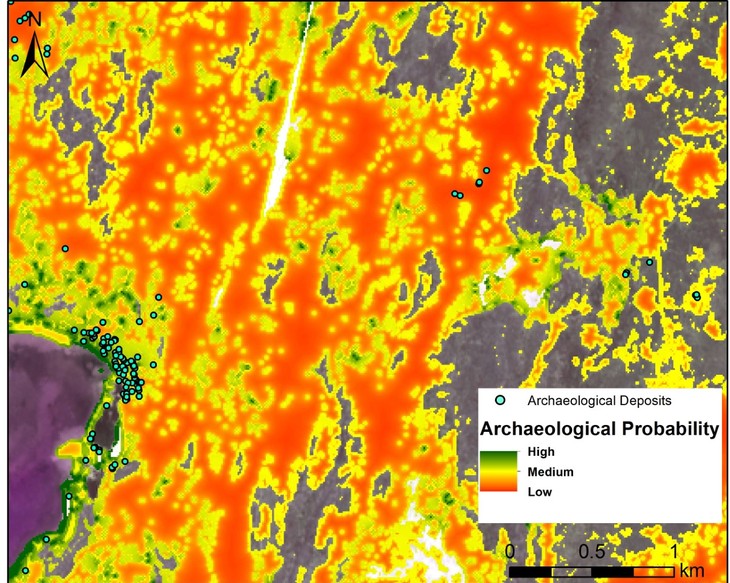
Computers scour satellite imagery to unveil Madagascar’s mysteries
Posted on February 19, 2020UNIVERSITY PARK, Pa. — Scientists may be a step closer to solving some of anthropology’s biggest mysteries thanks to a machine learning algorithm that can scour through remote sensing data, such as satellite imagery, looking for signs of human settlements, according to an international team of researchers.
In a study of satellite imagery taken of Madagascar, the team found evidence that suggests the island’s early inhabitants moved into areas and made the area more suitable for human inhabitation, said Kristina Douglass, assistant professor of anthropology, Penn State. This finding offers further evidence for a theory that predicts that as people move from resource-rich areas to less suitable sites, they tend to improve the land for their communities.
“We have a baseline assumption that people start in the most preferable areas with access to the best resources and, as that degrades and populations expand, they’ll move to less suitable areas,” said Douglass. “We actually found some evidence that there was a concentration in the medium suitability areas, which might be evidence of what ecologists and anthropologists refer to as an Allee effect, although this is something that we still have to confirm.”
She added that the Allee effect indicates that humans who live in these medium resource-rich areas may use various agricultural and land modification techniques to improve their surroundings.
“Over time, people are actually creating their landscape,” said Douglass. “They’re not degrading it necessarily. They’re creating a landscape that is preferable for people.”
The effects also show the importance of social ties and social networks, according to the researchers.
“Allee effects can also indicate that closely-knit social ties kept communities together, so when areas became overcrowded, large groups would resettle in new places,” said Dylan Davis, a doctoral student in anthropology at Penn State and an author of the study. “This means that in addition to creating the landscapes they lived on, ancient peoples were likely engaged in closely tied kinship and social networks.”
In addition to finding evidence for the Allee effect, Douglass said that the team also found that machine learning tools are uniquely suited to help scientists study Madagascar, which is the fourth largest island in the world and the center of perplexing archeological controversies.
“It is one of the places on earth that has generated a huge amount of debate in archeology about some pretty basic questions: When did people first get there, for example? How did all these species of large animals that used to live on the island go extinct, sometime in the last thousand years?” said Douglass.
She added Madagascar’s size and rugged terrain make archeological investigations expensive and time consuming.
“Archeologists have been working on Madagascar for the past 50 or 60 years and, yet, we still have little coverage overall of this very large island, in terms of sites that have been visited, regions that have been explored archeologically,” said Douglass. “We estimate that about 75% of the island has not been explored archeologically. Counter that with all these burning questions that have stimulated debate and have not been easy to answer, it’s not surprising that we’re getting stumped on some of these big questions.”

Davis said that this study is the first in over 20 years to use satellite images to try and locate archaeological sites on Madagascar.
“The fact is that these technologies are largely untapped and important sources of information for studying the history of the island,” she said.
The researchers’ machine learning algorithm, however, can examine satellite imagery from free sources to identify areas where human inhabitation is most probable. These sites might not look much different to human archeologists who look at the terrain in photographs, said Douglass, but by focusing their efforts on high probability sites identified by the algorithm, anthropologists could concentrate their time, money and effort.
“Using this method, we were able to systematically scan over 20 square miles of land per hour,” said Davis. “This exponentially increases the rate of survey and discovery of archaeological deposits when compared to traditional ground-based methods.”
Douglass said the algorithm might be able to help archeologists and anthropologists tackle even bigger — in fact, continent-sized — challenges. For example, the algorithm could help studies of Africa, which is a massive continent with rugged terrain and, as the cradle of human civilization, the center of important anthropological questions and debates.
The researchers used freely downloadable satellite imagery from the European Space Agency’s satellite. The resolution of the imagery is not refined enough to let humans detect signs of ancient human civilization, Douglass said, but the resolution is adequate for a predictive algorithm.
The team trained the algorithm using the best theoretical assumptions on how people settle areas. The algorithm compares those theoretical assumptions with the current landscape to select high, medium, or low probability areas of evidence of human inhabitation.
Remote sensing data can also come from non-satellite sources, including footage taken from drones.
Douglass said she is excited for future research, which may include access to satellite imagery that has higher resolution.
“With higher resolution images, we may be able to identify specific traces of cultural deposits, which would reduce the time spent on walking surveys even further,” said Davis.
Also working on the project were Vanillah Andriankaja, Tahirisoa Lorine Carina, Zafy Maharesy Chrisostome, Christophe Colombe, Felicia Fenomanana, Laurence Hubertine, Ricky Justome, François Lahiniriko, Harson Léonce, George Manahira, Briand Venance Pierre, Razafimagnefa Roi, Patricia Soafiavy, Faralahy Victorian and Vavisoa Voahirana, all of the Morombe Archaeological Project; and Barthélémy Manjakahery, of the Université de Toliara, Madagascar.
The team reported their findings in the Journal of Archaeological Science.
An Institute for Computational and Data Sciences seed grant, a Hill Fellowship Award and the PA Space Grant Consortium supported this work.
Share
Related Posts
- Featured Researcher: Nick Tusay
- Multi-institutional team to use AI to evaluate social, behavioral science claims
- NSF invests in cyberinfrastructure institute to harness cosmic data
- Center for Immersive Experiences set to debut, serving researchers and students
- Distant Suns, Distant Worlds
- CyberScience Seminar: Researcher to discuss how AI can help people avoid adverse drug interactions
- AI could offer warnings about serious side effects of drug-drug interactions
- Taking RTKI drugs during radiotherapy may not aid survival, worsens side effects
- Cost-effective cloud research computing options now available for researchers
- Costs of natural disasters are increasing at the high end
- Model helps choose wind farm locations, predicts output
- Virus may jump species through ‘rock-and-roll’ motion with receptors
- Researchers seek to revolutionize catalyst design with machine learning
- Resilient Resumes team places third in Nittany AI Challenge
- ‘AI in Action’: Machine learning may help scientists explore deep sleep
- Clickbait Secrets Exposed! Humans and AI team up to improve clickbait detection
- Focusing computational power for more accurate, efficient weather forecasts
- How many Earth-like planets are around sun-like stars?
- Professor receives NSF grant to model cell disorder in heart
- SMH! Brains trained on e-devices may struggle to understand scientific info
- Whole genome sequencing may help officials get a handle on disease outbreaks
- New tool could reduce security analysts’ workloads by automating data triage
- Careful analysis of volcano’s plumbing system may give tips on pending eruptions
- Reducing farm greenhouse gas emissions may plant the seed for a cooler planet
- Using artificial intelligence to detect discrimination
- Four ways scholars say we can cut the chances of nasty satellite data surprises
- Game theory shows why stigmatization may not make sense in modern society
- Older adults can serve communities as engines of everyday innovation
- Pig-Pen effect: Mixing skin oil and ozone can produce a personal pollution cloud
- Researchers find genes that could help create more resilient chickens
- Despite dire predictions, levels of social support remain steady in the U.S.
- For many, friends and family, not doctors, serve as a gateway to opioid misuse
- New algorithm may help people store more pictures, share videos faster
- Head named for Ken and Mary Alice Lindquist Department of Nuclear Engineering
- Scientific evidence boosts action for activists, decreases action for scientists
- People explore options, then selectively represent good options to make difficult decisions
- Map reveals that lynching extended far beyond the deep South
- Gravitational forces in protoplanetary disks push super-Earths close to stars
- Supercomputer cluster donation helps turn high school class into climate science research lab
- Believing machines can out-do people may fuel acceptance of self-driving cars
- People more likely to trust machines than humans with their private info
- IBM donates system to Penn State to advance AI research
- ICS Seed Grants to power projects that use AI, machine learning for common good
- Penn State Berks team advances to MVP Phase of Nittany AI Challenge
- Creepy computers or people partners? Working to make AI that enhances humanity
- Sky is clearing for using AI to probe weather variability
- ‘AI will see you now’: Panel to discuss the AI revolution in health and medicine
- Privacy law scholars must address potential for nasty satellite data surprises
- Researchers take aim at hackers trying to attack high-value AI models
- Girls, economically disadvantaged less likely to get parental urging to study computers
- Seed grants awarded to projects using Twitter data
- Researchers find features that shape mechanical force during protein synthesis
- A peek at living room decor suggests how decorations vary around the world
- Interactive websites may cause antismoking messages to backfire
- Changing how government assesses risk may ease fallout from extreme financial events
- Differences in genes’ geographic origin influence mitochondrial function
- ICS Affiliate named AGU fellow
- ICS co-sponsors Health, Environment Seed Grant Program